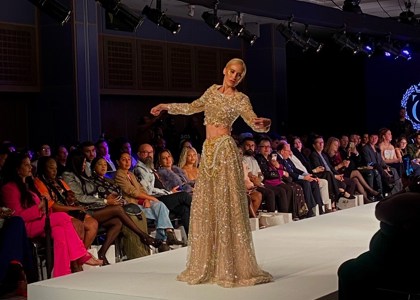
Take your first step towards you dream fashion career with the Fashion Retail Academy. From sharing opportunities like volunteering at London Fashion Week to real-world industry guidance, we're here to support your journey!
30th January 2025
Date 30th January 2023
As a new and expanding area of the fashion industry, job roles in retail analytics and forecasting are rapidly evolving and there are many opportunities for career progression. According to a recent study by Gov.uk, 46% of businesses hiring data analysts in the UK are struggling to recruit for available roles.
But what actually is data analytics for retail and how does it relate to the fashion industry? Read our ultimate guide and discover what retail analytics is, the benefits of using it within a fashion business, the different types of retail analytics and how to start a career as a retail data analyst.
Retail analytics is the practice of collecting, analysing and using data to inform and improve the performance of a retail business. It can be used to benefit many aspects of a retail business including merchandising, marketing and operations.
Retail analysis can help businesses better understand consumer behaviour and preferences, identify trends and patterns, optimise pricing and promotion strategies, and improve operations and supply chain management.
Data analytics for retail can also be used to measure the effectiveness of marketing campaigns, understand the impact of external factors on sales, and identify opportunities for growth and expansion.
There are lots of different advantages to using retail analytics for brands. Some of the main benefits of data analytics for retail include:
Retail analytics helps businesses make data-driven decisions that are based on real-time data and insights, rather than gut feelings or assumptions. This can lead to more effective and profitable business decisions.
Retail analysis also allows brands to make their marketing efforts more focussed. For example, campaigns that are aimed at a specific target audience are more likely to convert and show promising ROI in comparison to a campaign that attempts to appeal to the masses.
Retail analytics can provide brands with a much greater understanding of their customers. Not only in terms of age and gender but also in terms of shopping habits, preferences, hobbies and interests. This insight allows retailers to create more targeted and effective marketing campaigns.
For example, knowing what type of products their customers prefer, how much they would typically spend and what influences their decision making is invaluable for retailers.
It allows them to make personalised recommendations and promotions for each individual customer which can in turn increase conversions.
Data driven retail campaigns can also increase the efficiency of a brand's marketing activities. Knowing exactly who their customers are and how to communicate with them can make their efforts much more effective at increasing sales and ROI.
For example, some customers will be more receptive to Instagram ads whereas others may be more receptive to email marketing. Retail analytics ensures that retailers are utilising the right methods to increase conversions. Knowing which channel to communicate to their audience through is also an effective method of reducing unnecessary costs.
Retail analysis can help businesses identify trends and patterns that can inform future business decisions and allow them to respond more quickly to changes in the market.
Analysing spending habits and sales helps retailers identify their best and worst selling products. This allows them to make informed decisions about which products to put more stock behind and which ones to put on sale or promotion.
Retail analytics can help businesses better understand what their customers' pain points are. Addressing these pain points can increase customer satisfaction and loyalty.
For example, if customers consistently abandon their carts at certain points of the checkout process, this will be highlighted in the data. Therefore improving the checkout experience will not only improve customer experience and loyalty, but it could also increase sales too.
Overall, the main aim of retail analytics is to increase sales and revenue. By understanding their customers, brands can adapt their promotion strategies and improve their operations to provide a superior service to their competitors.
Doing so can help businesses achieve long-term success in a rapidly-changing market.
There are four key types of retail analytics that each play an important role in providing today’s retailers with key insights into their business operations.
The four types of retail analytics are;
Descriptive analytics is a type of data analytics that looks at past data to give an account of what has happened. It works by bringing in raw data from multiple sources such as Google Analytics, POS terminals, inventory systems, OMS and ERPs to generate valuable insights into past performance.
Techniques used in descriptive analytics include data visualisation, summarisation statistics (such as mean, median, mode, and standard deviation), and correlation analysis. Results are typically presented in reports, dashboards, bar charts and other visualisations that are easily understood.
Descriptive analytics can help to identify previous issues within a business. However it doesn’t explain why those previous issues have occurred unless combined with other types of data analytics that can show patterns and correlations, e.g. predictive analytics or prescriptive analytics.
Diagnostic analytics is a type of data analytics that looks at past data to give an account of why something has happened. Taking the same raw data used in descriptive analytics, diagnostic analytics uses statistical analysis, algorithms, and sometimes, machine learning, to drill deeper into the data and find correlations between data points.
Diagnostic analytics is particularly useful at highlighting anomalies and potential issues within a retail business that do not match pre-programmed benchmarks and business rules. This includes things such as technical issues and errors within an ecommerce website.
Historically this type of retail analysis used to be done manually but doing so can leave too much room for human error. This is why larger retailers use machine learning and AI to carry out diagnostic analytics.
Predictive analytics is a type of data analytics that makes predictions about future outcomes based on historical data. Predictive analytics uses statistical algorithms and machine learning to analyse historical sales data and other information to identify patterns and make predictions about future sales.
Retailers use predictive analytics to forecast demand for products, optimise pricing, manage inventory and create personalised marketing campaigns. Insights into customer behaviour, purchasing patterns and preferences can be used to predict which customers are likely to leave, which products are likely to be popular, and how much stock to put behind each product.
Although this type of retail analysis can be carried out manually using excel, the data is quite complex and it would be incredibly difficult to account for all the possible factors that could influence future sales. This is why most retailers use predictive analytics, which relies on AI and advanced mathematics to more accurately forecast future trends.
Prescriptive analytics is a type of data analytics that provides recommendations on how to improve business performance based on multiple predicted outcomes. Essentially, prescriptive analytics tells retailers what they should do next to get the best results.
Prescriptive analytics can be used to determine the best pricing strategy for a product, the most effective inventory management plan, or the optimal logistics plan for delivering goods to customers. It can also be used to optimise marketing campaigns, plan staffing schedules and assess potential investment opportunities.
The prescriptive analytics process typically involves a combination of optimisation techniques, simulation models and machine learning algorithms. The models will analyse historical data and present a range of potential solutions, ranked by potential performance, so that the decision maker can choose the most appropriate one.
Overall, prescriptive analytics provides actionable insights and recommendations that allows retailers to make better decisions and improve their operations.
Retail analytics tools help businesses identify and analyse customer data to provide insight into a variety of different areas including which items are selling well, when items sell most, and what demographics purchase which items. There are a number of retail analytics tools that can help retailers acquire this information including:
The retail space is incredibly competitive which is driving more businesses than ever to adopt and invest in technology in an effort to stand out from the crowd online and on the high street. With this in mind, the demand for retail analysts has also increased to ensure that every aspect of their retail operations is efficient and effective.
Retail analysts work in fast-paced environments where they are constantly crunching numbers and analysing data. They work closely with other members of the retail team, such as the marketing department, to ensure that the brand is meeting its sales goals.
The salary of retail analysts is also pretty competitive and they can expect to earn between £30,000 - £60,000, with room for progression.
If you love data, problem solving and have critical thinking skills, then a job as a retail analyst could be just for you. Start your career today by studying retail analytics at the Fashion Retail Academy.
To get started as a retail analyst, you’ll need to prove you have all the required skills. This means you’ll usually need to study a specialist course and have some work experience to boost your CV.
For most jobs, you normally need a Higher National Diploma (HND) or a degree in a relevant subject. At the Fashion Retail Academy, we offer a specialist Level 4 Data Analytics For Retail diploma, that will provide you with the skills and knowledge you need to start your career. Alternatively, for those preferring to study online or from home, we also offer an Online Data Analytics For Retail course.
Our Data Analytics for Retail courses will help you master the essential skills required for an entry-level position in the fashion industry. Not only will you learn data analysis skills that will help you identify valuable insights about customer behaviour but you’ll also learn how to use this information to influence the future direction of a fashion business.
Our Data Analytics for Retail courses are endorsed by M&S and will teach you the fundamentals of data analysis within the fashion retail industry. You’ll learn to identify and solve problems within a brand, as well as discover how to interpret trends and highlight new opportunities to maximise the growth of a business.
During the Data Analytics for Retail course, you'll complete 4 projects that cover a range of key topics including:
Throughout our retail analytics course, you'll have regular seminars, talks and masterclasses from our brand partners. We’ll help you develop a strong industry network that will support you throughout your career. You’ll also take part in projects and challenges set by brands that help you to develop valuable, practical skills. In addition, you will also have the opportunity to take part in a 3 week industry placement.
If you have any more questions about any of the courses on offer at The Fashion Retail Academy, send us an email to info@fra.ac.uk - we’d love to hear from you!
Read our guide How To Get Started In The Fashion Industry for more information.
Think you’ve got what it takes to become a Retail Data Analyst? Start your career today and study Data Analytics For Retail.
Take your first step towards you dream fashion career with the Fashion Retail Academy. From sharing opportunities like volunteering at London Fashion Week to real-world industry guidance, we're here to support your journey!
30th January 2025
This week Andrew Pakes MP visited the Fashion Retail Academy at Electra House and met with our Principal, Lee Lucas.
27th January 2025
At just 16, Ellie took a bold step towards her future, enrolling on our Level 3 Fashion Retail Diploma. From live projects with major brands to becoming the face of the FRA as a Student Marketing Assistant, Ellie's journey highlights the incredible opportunities waiting for you at the FRA. Ready to start your journey?
23rd January 2025